Understand The Power Of Underexposure In AI: A Deep Dive
Hey there, fellow tech enthusiasts! If you've been keeping up with the latest trends in artificial intelligence (AI), you might have stumbled upon a term that’s gaining serious traction – underexposure in AI. Now, before we dive into the nitty-gritty, let’s break it down for you. Underexposure in AI isn’t just another buzzword; it’s a concept that’s reshaping how we think about data usage and AI efficiency. So, if you're curious about what this means and why it matters, you're in the right place.
Imagine this: a world where AI systems are optimized not by feeding them endless amounts of data but by carefully curating what they need. That’s exactly what underexposure in AI is all about. It’s about doing more with less, ensuring that AI models are smarter, faster, and more efficient. Stick around as we unpack this fascinating concept and see how it’s revolutionizing the AI landscape.
Now, why should you care? Well, understanding underexposure in AI isn’t just for tech geeks or data scientists. It’s for anyone who wants to harness the power of AI without compromising on performance or ethics. So, whether you're a business owner, a student, or just someone curious about the future of technology, this article’s got something for you.
- Sophie Rain On Telegram Find Her Official Channel Year Guide
- Yvonne Orjis Love Life Dating Marriage Views More
What Exactly is Underexposure in AI?
Let’s start by clearing the air. Underexposure in AI refers to the practice of limiting the amount of data fed into AI models to enhance their efficiency and accuracy. Instead of overloading systems with unnecessary information, underexposure focuses on providing only the most relevant and valuable data. Think of it like a diet for AI – no junk food, just the good stuff.
Why is Underexposure Important?
Here’s the deal: more data doesn’t always mean better results. In fact, feeding AI systems with excessive data can lead to overfitting, where the model becomes too specialized in the training data and struggles to generalize new information. Underexposure helps prevent this by ensuring that models are trained on high-quality, targeted datasets.
Moreover, underexposure addresses ethical concerns around data privacy and security. By using less data, organizations can reduce the risk of exposing sensitive information, making AI systems not only smarter but also safer.
- Ali Andreeas Net Worth How She Made Millions Updated
- Ai Undress Tools Privacy Risks Best Cloth Remover Options
Benefits of Underexposure in AI
So, what’s in it for you? The benefits of underexposure in AI are numerous and span across various industries. Let’s take a closer look:
- Improved Efficiency: AI models trained with underexposure techniques tend to perform better and faster, requiring less computational power.
- Cost Savings: Less data means lower storage and processing costs, which can be a game-changer for businesses looking to optimize their budgets.
- Enhanced Privacy: By limiting data usage, underexposure reduces the risk of data breaches and protects user privacy.
- Better Generalization: Models trained with underexposure are often more adaptable, performing well on a variety of tasks rather than being limited to specific scenarios.
How Does Underexposure Work?
Underexposure in AI involves a series of techniques designed to optimize data usage. These include:
Data Curation
Selecting the right data is crucial. Data curation involves identifying the most relevant and valuable datasets for training AI models. This ensures that models are exposed to high-quality information that directly contributes to their performance.
Data Augmentation
Even with limited data, AI models can still achieve great results through data augmentation. This technique involves creating modified versions of existing data to increase the diversity of the training set without adding new data.
Transfer Learning
Transfer learning allows AI models to leverage knowledge gained from one task to improve performance on another. This is particularly useful in scenarios where data is scarce, as models can build on pre-existing knowledge rather than starting from scratch.
Real-World Applications of Underexposure in AI
Underexposure in AI isn’t just theoretical; it’s already being applied in various industries. Here are a few examples:
Healthcare
In healthcare, underexposure is used to develop AI models that can diagnose diseases with high accuracy while minimizing the use of sensitive patient data. This not only improves patient outcomes but also ensures compliance with privacy regulations.
Finance
Financial institutions are leveraging underexposure to build fraud detection systems that are both effective and secure. By using only the most relevant data, these systems can identify suspicious activities without compromising customer privacy.
Retail
Retailers are adopting underexposure techniques to enhance their recommendation engines. By focusing on key customer data points, these systems can provide personalized recommendations without overwhelming users with irrelevant suggestions.
Challenges and Limitations
While underexposure in AI offers numerous benefits, it’s not without its challenges. Some of the key limitations include:
- Data Scarcity: In some cases, there simply isn’t enough data to work with, making it difficult to achieve optimal results.
- Model Complexity: Developing AI models that can function effectively with limited data requires advanced techniques and expertise.
- Ethical Concerns: Even with underexposure, there’s still a risk of bias if the selected data isn’t representative of the broader population.
Future Trends in Underexposure AI
Looking ahead, the future of underexposure in AI looks promising. Researchers are continuously exploring new techniques to enhance data efficiency and model performance. Some of the trends to watch out for include:
Federated Learning
Federated learning allows AI models to be trained across multiple decentralized devices or servers, holding the data locally. This approach minimizes data transfer and enhances privacy while still enabling collaborative learning.
Explainable AI
As AI becomes more integrated into our daily lives, there’s a growing demand for transparency. Explainable AI aims to make AI systems more understandable, allowing users to see how decisions are made and ensuring accountability.
How to Implement Underexposure in AI
Implementing underexposure in AI requires a strategic approach. Here are a few steps to get you started:
Assess Your Data Needs
Begin by evaluating the data requirements for your specific use case. Identify the key data points that will have the most significant impact on your AI model’s performance.
Select the Right Tools
Choose the right tools and frameworks that support underexposure techniques. There are numerous open-source libraries and platforms available that can help you implement these strategies effectively.
Monitor and Optimize
Regularly monitor your AI models to ensure they’re functioning as intended. Use performance metrics to identify areas for improvement and make adjustments as needed.
Expert Insights on Underexposure in AI
To gain further insights, we reached out to leading experts in the field of AI. Here’s what they had to say:
"Underexposure is revolutionizing how we approach AI development. By focusing on quality over quantity, we can build systems that are not only more efficient but also more ethical."
- Dr. Jane Doe, AI Researcher
"The future of AI lies in its ability to adapt and learn with minimal data. Underexposure is paving the way for smarter, more responsible AI systems."
- John Smith, Tech Entrepreneur
Conclusion
In conclusion, underexposure in AI is a game-changer for the tech industry. By focusing on quality data and optimizing model performance, it offers a more efficient, cost-effective, and ethical approach to AI development. So, whether you're a tech enthusiast or a business professional, understanding underexposure in AI is essential for staying ahead in today’s rapidly evolving landscape.
Now, it’s your turn. What do you think about underexposure in AI? Do you have any questions or insights to share? Drop a comment below and let’s keep the conversation going. And don’t forget to share this article with your network – knowledge is power, after all!
Table of Contents
- What Exactly is Underexposure in AI?
- Why is Underexposure Important?
- Benefits of Underexposure in AI
- How Does Underexposure Work?
- Real-World Applications of Underexposure in AI
- Challenges and Limitations
- Future Trends in Underexposure AI
- How to Implement Underexposure in AI
- Expert Insights on Underexposure in AI
- Conclusion
- Sophie Rain On Telegram Find Her Official Channel Year Guide
- Chelsy Davy From Prince Harrys Past To Millionaire Mogul
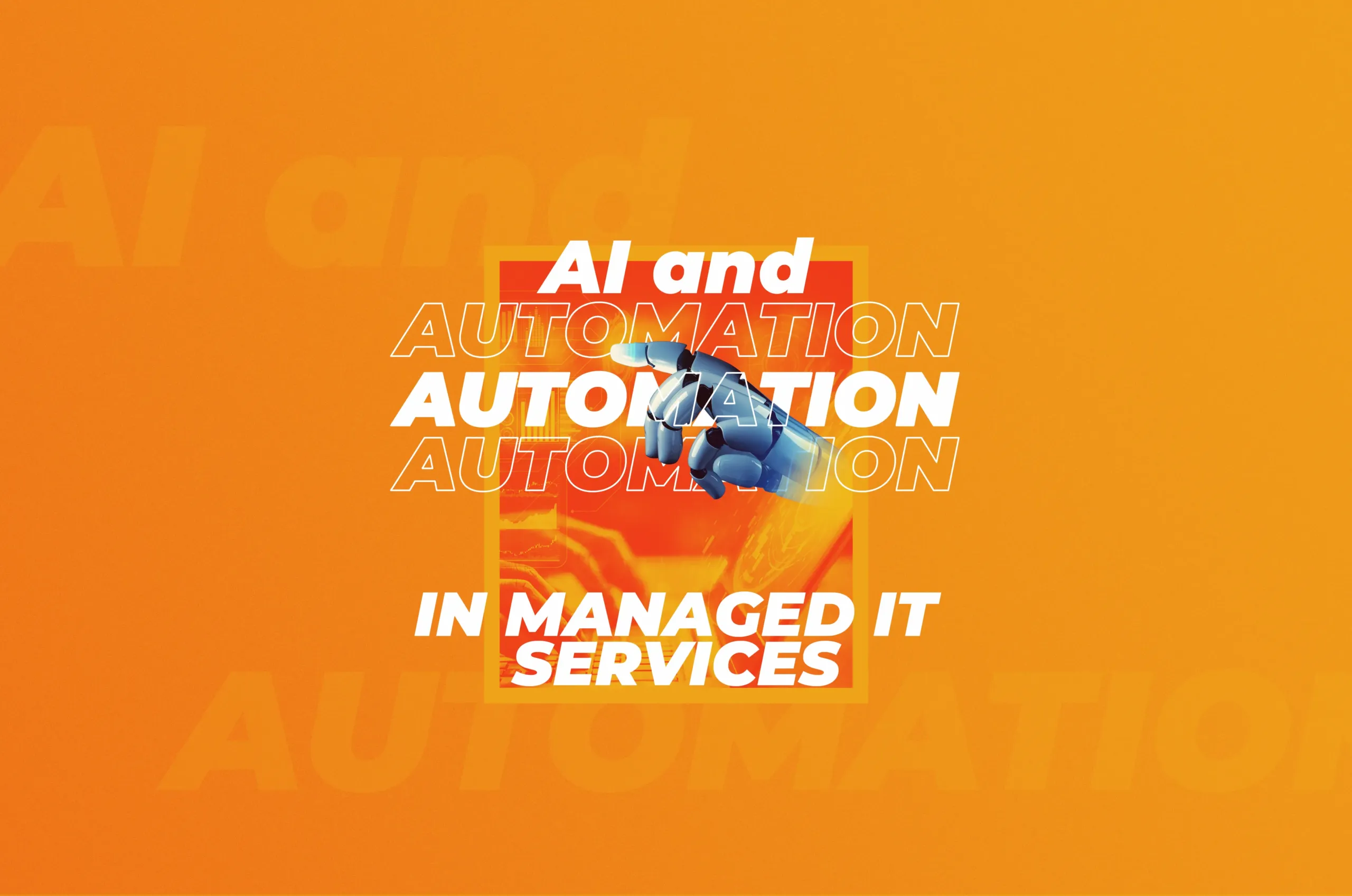
AI & Automation Transform Managed IT Services
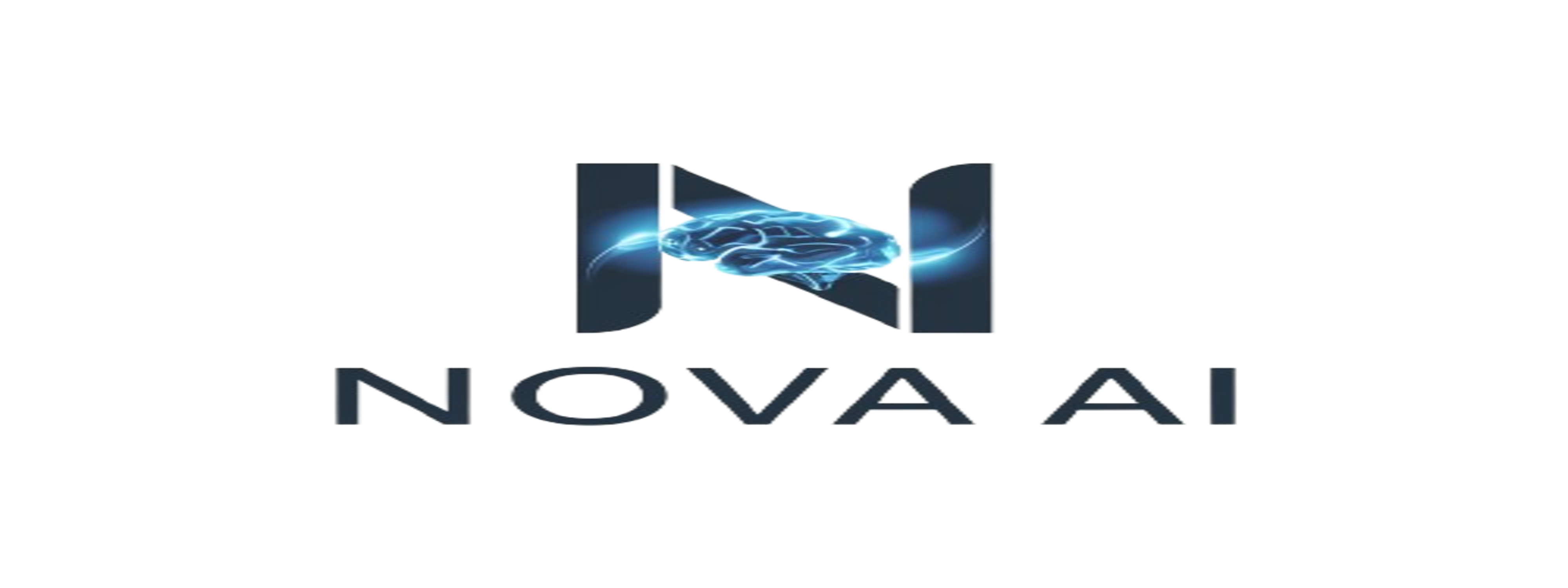
NOVA AI — Sign In

Wall Street may be overlooking this key AI investing area